01. 量子計算基礎

Target Audience: High school & college students, beginners in quantum computing
Duration: 1 week (5 days, 3–4 hours per day)
Format: Lectures, hands-on simulations, discussions, and mini-projects
Prerequisites: Basic knowledge of algebra and probability; no prior quantum experience required
Day 1: Introduction to Quantum Computing
• What is Quantum Computing? Overview and historical context
• Classical vs. Quantum Computing: Key differences
• Core Concepts: Superposition, Entanglement, and Quantum Parallelism
• Introduction to Qubits: Bloch sphere representation
• Hands-on Activity:
• Use IBM Quantum Experience or a Python-based quantum simulator (Qiskit) to create and visualize qubits
Day 2: Quantum Gates and Circuits
• Quantum Gates vs. Classical Gates
• Basic Quantum Gates: Pauli-X, Y, Z; Hadamard (H); Phase (S, T); CNOT
• Building a Simple Quantum Circuit
• Quantum Measurement: How quantum states collapse
• Hands-on Activity:
• Implement basic quantum circuits using Qiskit
• Visualize circuit operations using quantum circuit diagrams
Day 3: Quantum Algorithms & Computation
• Quantum Speedup & Complexity: Why quantum computers are powerful
• Key Quantum Algorithms:
• Deutsch-Jozsa Algorithm (Introduction to quantum advantage)
• Grover’s Search Algorithm (Faster search)
• Hands-on Activity:
• Simulate Grover’s Algorithm in Qiskit
• Explore circuit execution on a real quantum processor (IBM Quantum)
Day 4: Quantum Cryptography and Applications
• Quantum Cryptography Basics:
• BB84 Protocol: Secure quantum key distribution
• Shor’s Algorithm: Breaking RSA encryption
• Quantum Applications:
• Optimization (e.g., portfolio optimization in finance)
• Machine learning (quantum-enhanced AI)
• Drug discovery & materials science
• Hands-on Activity:
• Simulate BB84 encryption protocol using Qiskit
Day 5: Future of Quantum Computing & Mini Project
• Current State of Quantum Hardware: IBM, Google, Rigetti, and others
• Quantum Error Correction & Noise: Challenges in building scalable quantum computers
• Future Prospects: Quantum AI, Quantum Internet
• Mini Project:
• Students choose one:
• Create a quantum circuit solving a simple problem
• Simulate a quantum teleportation protocol
• Implement a basic quantum algorithm (e.g., Grover’s search)
• Final Presentations: Showcase project results and discuss key learnings
Course Outcome:
By the end of the course, students will:
Understand quantum computing fundamentals and key principles
Gain hands-on experience with Qiskit and quantum circuit simulations
Learn about quantum algorithms and real-world applications
Complete a small quantum computing project
02.人工智慧(AI)基礎知識
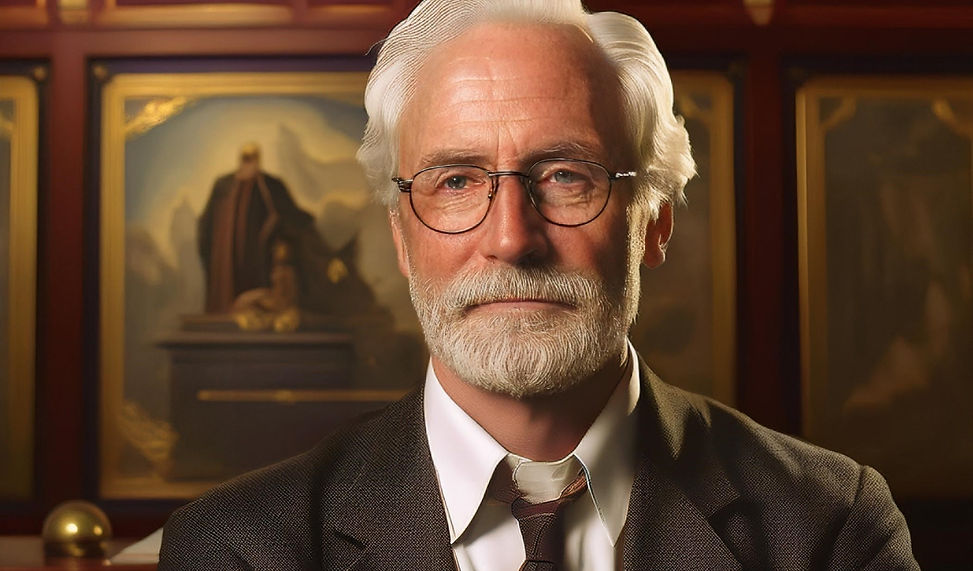
目標對象:高中生、大學生、人工智慧初學者
持續時間:1週(5天,每天3-4小時)
形式:講座、動手編碼、小組討論和專案工作
先修條件:基本程式設計知識(推薦 Python)
第一天:人工智慧與機器學習簡介
• 什麼是人工智慧?歷史、範圍和應用
• 人工智慧的類型:狹義人工智慧與廣義人工智慧
• 機器學習 (ML) 簡介:監督式學習、無監督學習和強化學習
• 人工智慧倫理與偏見:理解人工智慧模式中的公平性
• 實作活動:
• 在 Google Colab 中執行一個簡單的 AI 模型(例如,使用 TensorFlow/Keras 訓練一個小型影像分類器)
第二天:數據與人工智慧——基礎
• 資料在人工智慧中的重要性:資料收集、清理和預處理
• 資料型態:結構化資料與非結構化資料
• 特徵工程與選擇
• 實作活動:
• 使用 Python(Pandas、NumPy)處理和清理資料集
• 使用 Matplotlib/Seaborn 探索資料集以進行視覺化
第三天:監督學習-訓練人工智慧模型
• 監督學習基礎:迴歸與分類
• 常見演算法:
• 線性迴歸
• 決策樹
• 神經網路(深度學習簡介)
• 實作活動:
• 使用 Scikit-Learn 訓練一個簡單的分類器(例如,使用 MNIST 資料集識別手寫數字)
第四天:神經網路與深度學習
• 什麼是神經網路?感知器和多層網路的基礎知識
• 深度學習簡介:卷積神經網路 (CNN)、循環神經網路 (RNN)
• 實作活動:
• 使用 TensorFlow/Keras 訓練 CNN 對影像進行分類
• 使用 Google Teachable Machine 等互動式 AI 工具
第五天:人工智慧應用與小型項目
• 現實世界應用中的人工智慧:醫療保健、金融、機器人、創意人工智慧
• 人工智慧的未來:生成式人工智慧、量子人工智慧
• 迷你專案:
• 學生選擇以下之一:
• 建立一個基本的 AI 聊天機器人
• 在文字資料上訓練情緒分析模型
• 開發影像分類器
• 最終示範:展示專案成果與關鍵學習內容
課程成果:
課程結束時,學生將:
了解核心 AI 和 ML 概念
獲得 Python、Scikit-Learn 和 TensorFlow 的實務經驗
學習預處理資料並建立簡單的人工智慧模型
完成一個小型AI項目